Introduction Diverse imaging modalities exist for evaluation of stroke. Since its first application in brain Magnetic Resonance Imaging (MRI), diffusion weighted imaging (DWI) became one of the essential and optimal techniques for diagnosing acute ischemic stroke1,2. DWI exhibits tissue characteristics by providing information of the random motion of the water molecules, so-called Brownian movement in
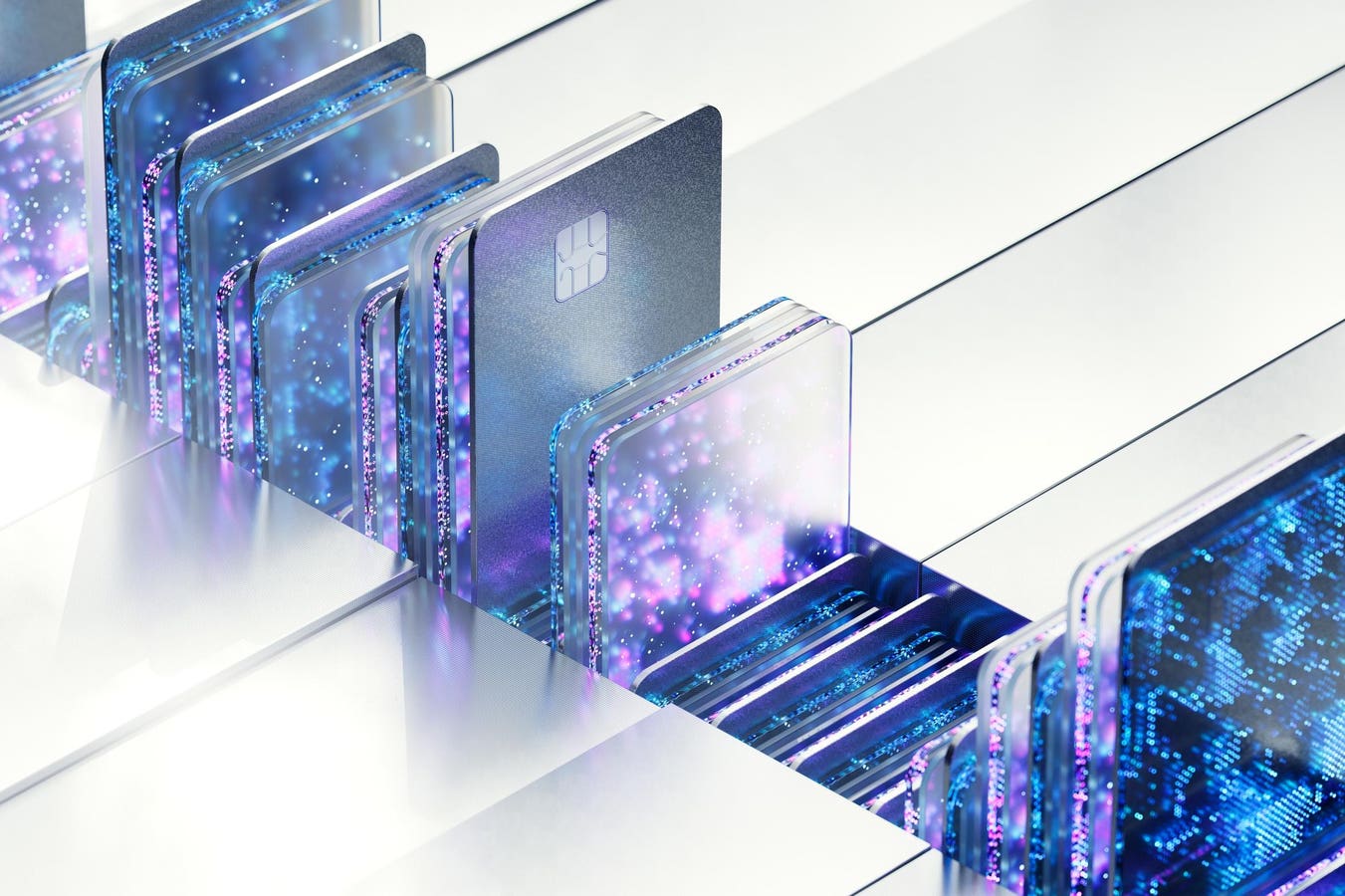
Fintech Meets AI: Key Concepts Of A Data-Driven Financial Revolution – Forbes
Bhavdeep Sethi is a leader in AI-powered fintech and Founding Member at Frec.
When it comes to artificial intelligence (AI), there’s plenty to unpack—machine learning (ML), deep learning and natural language processing (NLP) are all playing transformational roles in how fintech companies handle decision-making and automation at scale. But adopting these technologies isn’t without its challenges. Issues like transparency, financial data quality and regulatory compliance must be addressed for fintech to fully harness AI in a sustainable, secure way. This article explores some key AI applications in fintech, highlighting both the opportunities and risks they bring to the table.
Machine Learning: Driving Decision-Making At Scale
Machine learning (ML) is the beating heart of AI in fintech, enabling systems to identify patterns and make data-driven predictions.
Example: Credit Scoring
Traditional credit scoring models rely on a limited set of variables—like income and credit history—to assess risk. ML models, on the other hand, can consider additional granular data points such as recurring bill payments and spending patterns, allowing for more dynamic assessments of creditworthiness. This leads to more accurate and comprehensive risk assessments, but the complexity of ML models introduces transparency challenges—often referred to as the “black box problem.” These opaque decision-making processes pose significant risks in highly regulated industries like finance, where transparency is critical for compliance and customer trust.
Key Concept: Explainable AI (XAI)
To address the challenge of transparency, explainable AI (XAI) tools like generalized additive models (GAMs) offer a way to combat the black box problem by breaking down how decisions are made. In a credit scoring system, for instance, GAMs can quantify how consistent bill payments influence the score and to what extent, making the model more interpretable for both customers and regulators. This increased transparency will continue to be critical in meeting regulatory requirements and ensuring fairness in AI-driven financial decisions.
Deep Learning: Tackling Complex Financial Patterns
Deep learning, a powerful subset of ML, excels at processing highly complex datasets and uncovering nonlinear patterns, making it perfect for market predictions and portfolio optimization.
Example: Portfolio Optimization
Deep learning models can analyze historical market trends, asset prices and real-time financial data to optimize portfolio allocations. These models identify correlations that traditional methods might miss, offering dynamic adjustments in response to market fluctuations. However, deep learning requires significant processing power which can make these models prohibitive to train and maintain. To perform effectively, they also need vast amounts of data, which can be a high barrier for firms with limited resources or constrained compute power.
Key Concept: Transfer Learning
Transfer learning addresses data scarcity and resource limitations by allowing a model trained on one task (e.g., stock market prediction) to apply its learned knowledge to another task, such as bond market analysis. Some models have been trained across stock markets of different countries with impressive results. By reusing pretrained models, fintech firms can deploy deep learning across different financial applications without needing massive computational resources, expanding AI’s potential even for companies with limited data.
Natural Language Processing: Making Sense Of Unstructured Data
Natural language processing (NLP) allows fintech companies to extract actionable insights from unstructured data sources like news articles and transcribed earnings calls.
Example: Sentiment Analysis
NLP-powered sentiment analysis is very commonly used to gauge public sentiment by scanning financial news, social media and company reports. By identifying shifts in public opinion, these systems can provide early warnings of market movements, allowing investors to make informed decisions. However, NLP models face challenges in accurately interpreting language nuances and contextual subtleties, which can lead to flawed predictions. Additionally, bias in the training data can influence results, particularly when it comes to sentiment analysis.
Key Concept: Transformer Models
Transformer models like BERT and GPT have significantly advanced NLP by processing text in context, making sentiment analysis more precise than previous methods. However, transformers are not immune to bias. While some of these issues may be mitigated with further iterations, fintech firms must still carefully and independently curate training data, and continuously monitor model outputs, to ensure fairness and reliability.
Anomaly Detection: Catching Outliers And Irregularities
Anomaly detection is a classic application of AI in fintech, helping institutions spot deviations from expected behaviors. While often associated with stock market predictions and opportunist market plays, it is more widely used to highlight unusual activities across various financial systems, including payments, trading and regulatory compliance.
Example: Fraud Detection
Traditional fraud detection models were given data to analyze from a single institution, which limited their ability to identify larger trends and complex, cross-institutional schemes. Privacy regulations prevented financial institutions from sharing raw data containing private customer information, leaving fraud detection models with narrow datasets, which could miss sophisticated patterns or repeat offenders.
Federated Learning: Collaborative Anomaly Detection
Federated learning offers a solution by allowing institutions to collaboratively train anomaly detection models without sharing sensitive data. Each institution trains a local model on internal data, sharing only encrypted model updates, not the actual transaction details. This results in a global model that can detect anomalies across a much broader dataset, improving accuracy while preserving privacy.
Navigating The Opportunities And Risks Of AI In Fintech
While exciting, firms must first fully learn the limitations and risks of these technologies as they work to hone their “AI edge.” Successfully navigating and implementing AI in fintech will require a disciplined approach, a deep understanding of each tool’s benefits, and careful attention to the risks these innovations introduce.
Forbes Technology Council is an invitation-only community for world-class CIOs, CTOs and technology executives. Do I qualify?