Introduction Diverse imaging modalities exist for evaluation of stroke. Since its first application in brain Magnetic Resonance Imaging (MRI), diffusion weighted imaging (DWI) became one of the essential and optimal techniques for diagnosing acute ischemic stroke1,2. DWI exhibits tissue characteristics by providing information of the random motion of the water molecules, so-called Brownian movement in
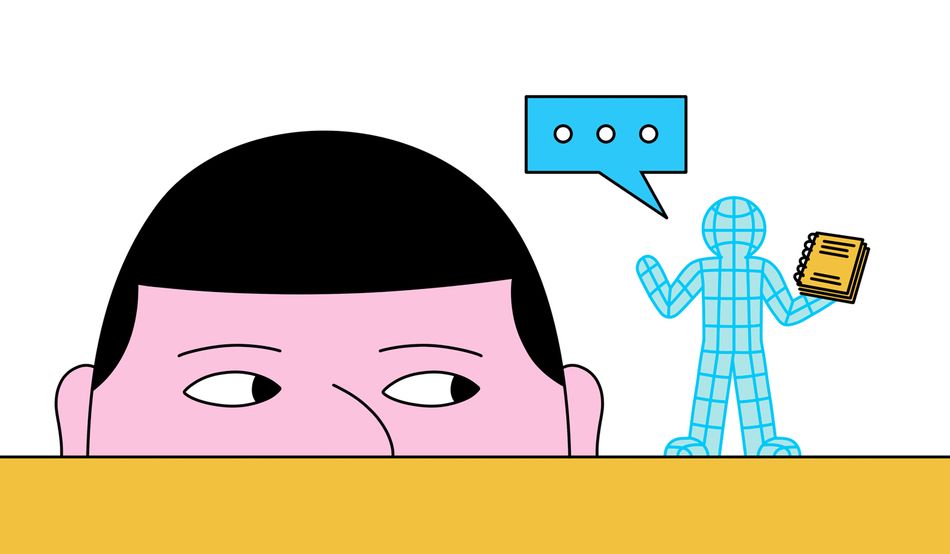
The Amish approach to AI – Prospect Magazine
As a young internet scholar, I was once asked to give a talk at a conference in the small town of Kalocsa, Hungary. The conference was called “The Internet: By 2015, It Will Be Entirely Unavoidable”, a title that has proven to be accurate, if not exactly a strong sales pitch.
I’ve thought back to that conference while examining my feelings as a technology commentator in the age of AI. Whether or not I want to write about artificial intelligence, it seems entirely unavoidable. My beat is now this complex, enormously promising, clearly overhyped, but likely transformational set of technologies.
The unavoidability of AI became more acute this autumn, as the Royal Swedish Academy of Sciences gave two prizes to AI innovators. Geoffrey Hinton and John Hopfield received the 2024 Nobel Prize in Physics for their early work on neural networks, a method of machine learning that mimics how neurons connect in the human brain. The prize was not without controversy: neither Hinton nor Hopfield identify as physicists and Hinton admitted that the prize might indicate that it is time for the Nobel Committee to award in computer science.
I find the Nobel in Chemistry even more interesting. Two of three recipients work for DeepMind, the AI subsidiary of Google that has produced AlphaFold, a system that uses machine learning to predict the structure of proteins from their chemical sequences.
Structural biologists, the scientists most directly affected by AlphaFold, have taken pains to explain that the system, while incredibly impressive, has not “solved” protein folding. Writing in a top journal of the field, five biologists argue that, while the system “is astonishingly powerful”, biologists will still need to synthesise and experiment with proteins to see whether they are stable and safe enough to be promising drug candidates.
As AI transforms fields, there is a wave of critical reaction emphasising its limitations. I’ve been part of this wave, using this column to remind people that just because generative AI writes human-sounding paragraphs, those paragraphs are not necessarily true, accurate or reliably sourced. These objections, and the objections of the structural biologists, reveal parallel threads of excitement and deep discomfort with the rapid changes our fields are experiencing.
I had an “astonishingly powerful” moment with an AI system recently, experimenting with Google’s NotebookLM system. “LM” stands for “language model”, and there’s been enormous enthusiasm for “large language models” (LLMs)—massive AI systems that extrapolate from billions of documents to predict what words should come after a given phrase. Large language models generate realistic-seeming simulacra of human-authored text, and power systems such as ChatGPT. But LLMs are often unreliable: they “imagine” facts that have never occurred, cite sources that have never existed and present these “hallucinations” in convincing sounding prose.
NotebookLM works differently. It uses a massive LLM to create natural-seeming text, but it draws on a set of documents the user specifies to find its facts. I fed NotebookLM a long academic article my lab produced about our calculations of the size of YouTube, adding in some press pieces about our discoveries. NotebookLM quickly produced several remarkable documents. It offered a well-written abstract of the paper and suggested questions I could ask the model to learn more about the documents it had summarised. It generated a ten-minute long podcast featuring male and female hosts discussing our research, complete with cheesy jokes.
As I interrogated the system, I discovered two things that delighted and disturbed me. When I asked the system, “How did the authors discover the size of YouTube?”, the response generated was carefully footnoted, referencing only the documents I asked the system to investigate. When I asked NotebookLM questions that could not be answered by those papers, it admitted its limits. I asked it whether snakes are amphibians, an easy question for a large language model like ChatGPT. NotebookLM demurred, telling me the documents in its notebook were about social media, not biology.
That admission of limitations has forced me to take NotebookLM more seriously than tools like ChatGPT. I’ve told students that they are welcome to use ChatGPT to brainstorm ideas or to summarise documents, but warned them that it cannot be trusted for accuracy. NotebookLM, on the other hand, might be a system I could grow to rely on. And that’s deeply uncomfortable for me.
As a scholar and a journalist, one of the great pleasures of my life is reading enormous piles of documents and synthesising what I’ve learned. It’s possible that NotebookLM is as good—and lots faster—at this than I am.
In other words, like the structural biologists, I am feeling a combination of excitement about how I might use this tool to advance my own work and deep anxiety about whether AI is coming for my job. And that, in turn, got me thinking about the Luddites.
The much-derided Luddites were not simple-minded idiots who misunderstood and feared the machines brought into English factories. Instead, they were savvy labour activists at a moment when unions were illegal in Britain. Their attacks on machines were not indiscriminate, but focused on specific appliances they saw as threatening the livelihood of proud, skilled labourers.
It’s not hard to offer a neo-Luddite argument against large language models. My years of online writing represent some tiny fraction of the massive corpus of text used to generate the models used by NotebookLM to sound like a competent scholar. Perhaps I could be forgiven the desire to smash some machinery that threatens my livelihood. Instead, I find myself thinking of communities like the Amish who’ve set limits on what technology they embrace.
The Amish, a religious group in the United States, are well known for their decision not to adopt some common technologies, including the automobile and electricity in the home. Their use of horse and buggy makes them a visible example of resistance to modernity in some rural American communities.
Technology critic Kevin Kelly warns us not to think of the Amish as being stuck in the 1600s. Indeed, Amish woodworking workshops have sophisticated pneumatic tool systems that allow crafting of furniture at industrial scales. The Amish have a thoughtful and careful evaluative process for how they adopt technologies. Church elders discuss whether a new technology is likely to impact family, community and spiritual life. This leads to experiments and compromises.
Phones are used by the Amish, but not kept in the home where they could disturb the rhythms of daily life. Instead, they remain in phone sheds where one could go to transact business. As mobile phones began replacing wired phones, some Amish communities experimented with these, but only for use in buggies, creating a mobile phone-shed. Rather than a modern tendency to adopt new technologies when presented with them, the Amish practice—Kelly argues—is to evaluate carefully before adopting.
Academics have a lot to learn from this practice. We may have been comfortable warning our students away from LLMs as they generated unverifiable nonsense that could not pass academic scrutiny. But my experiments with NotebookLM created texts that would get reasonably high grades from me if a graduate student had used it to summarise papers into a literature review. This means I need to think carefully about this technology and how it might transform my work.
If the job of scholars is to produce as much knowledge as possible, perhaps these small language models that can competently summarise literature are an indispensable new tool in our arsenal. Yet another part of our job is to train a next generation of scholars to read carefully, synthesise and build on existing knowledge. It is certainly possible that teaching students to use NotebookLM rather than developing these skills themselves may create a generation of scholars less capable of this careful synthesis. Besides, if I become dependent on a tool like NotebookLM, will I lose this ability to make unexpected connections between bits of unrelated information? I don’t know, and I am more than a little scared.
Technological change feels relentless. The massive publishing, film and creative industries have thus far been unable to prevent AI companies from siphoning in billions of dollars of “intellectual property”. Do we as scholars and writers have any hope of slowing the progress of these systems? Can we have an Amish-inspired conversation about what we want these technologies to do for us, and if the transformations on the way are worth the cost?