Introduction Thyroid nodules are defined as space-occupying lesions within the thyroid gland that can be detected through imaging studies, differentiated from the surrounding thyroid tissue. These nodules can be benign or malignant, and according to recent epidemiological studies, up to 60% of the population have thyroid nodules1, with a malignancy rate of approximately 1–5%2. Further

Lighting the Way for Machine Vision and Deep Learning System Success | ASSEMBLY
While robots garner much of the attention in the industrial automation space, machine vision remains an indispensable technology for businesses of all types around the world, due to their ability to analyze images, extract useful data, and make decisions as part of a process.
As end user needs expand and change overtime, machine vision technology providers have responded with technology that takes machine vision systems to new heights, including deep learning technology. While subject to overhype when it first burst onto the scene in full force, deep learning has settled in to become a valuable tool in the realm of industrial automation. Still, as is the case with machine vision, systems leveraging deep learning software need high quality images to achieve success, which requires an optimal system setup.
Automating Amidst Labor Woes
In 2024, the global manufacturing economy was expected to see minimal growth of 0.6%, according to market intelligence company Interact Analysis. The long-term outlook offers more optimism, however, as Interact Analysis predicts improvements in 2025, continuing out to 2028. Still, as of September 2024, U.S. Bureau of Labor Statistics figures show that 7.4 million job openings exist in the United States, while 6.8 million people are out of work, showing that even if all unemployed people in the U.S. found work, more than half a million jobs would remain open.
Amidst this persisting labor shortage, industrial automation remains critically important for manufacturers looking to remain competitive now and into the future. It stands to reason then that technologies such as machine vision and artificial intelligence (AI) will see an uptick in adoption in the coming years. In fact, a number of reports suggest just that. From 2022 to 2028, the machine vision market is expected to see growth of about 6.4%, fueled by an influx of new applications as well as new vendors. AI also continues to gain steam in the industrial space, as 72% of respondents in a McKinsey AI survey indicate they have adopted AI in at least one business function.
Manufacturers today have access to more industrial automation tools—at more affordable costs—than ever before, and their capabilities continue to grow. Deep learning, an AI subset, has become a particularly valuable tool in the machine vision arsenal. No matter how powerful machine vision systems and their subcomponents and technologies have become, however, all systems still require proper specification, design, and setup. Without proper lighting, for instance, obtaining high quality images is not possible.
Optimal Machine Vision Lighting Examples
Different types of machine vision applications require different lighting setups. An optimal lighting setup must highlight the features to be detected relative to the background and must help create repeatable images regardless of part or product variation. Figure 1, for example, shows the same part with two different lighting setups. On the left, is a “good” image of a beveled gear captured with a dark field ring light, while the image on the right shows an image captured with a linear dark field setup, which produced linear effects on two sides of the gear. The image on the left shows sufficient contrast, allowing the software to clearly see teeth of the gear, along with the character printed directly on the part.
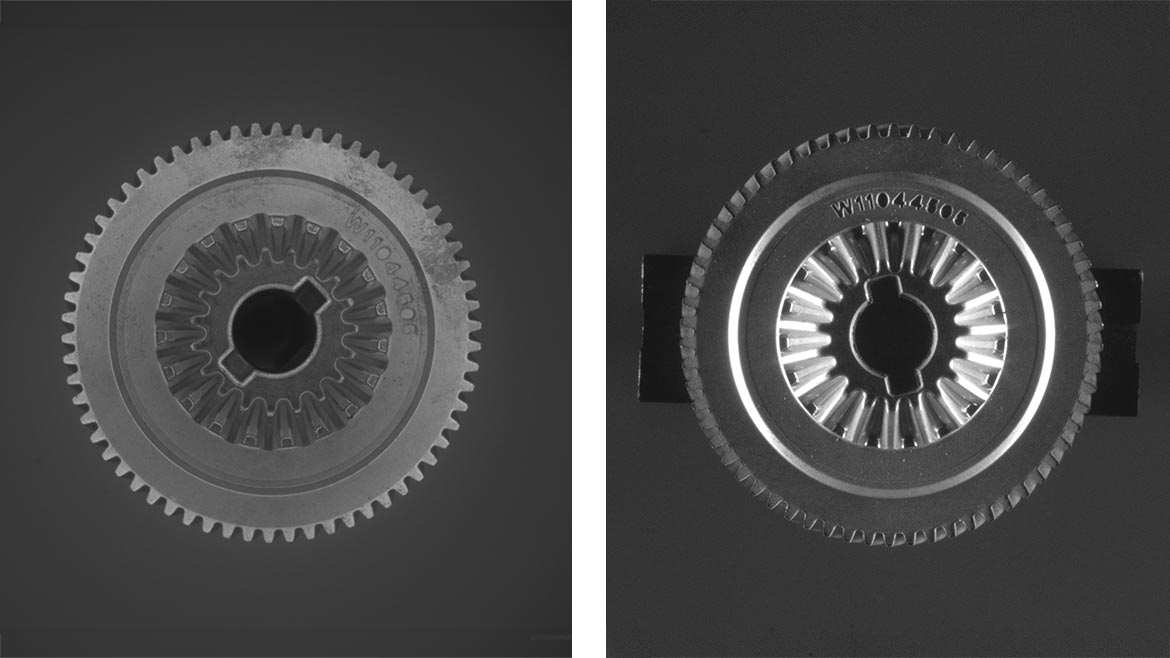
Some additional examples of common machine vision applications and their optimal lighting include:
Gaging and measurement: Many processes today require precise measurements of parts or products, or the distance between two or more points on objects. For gaging and measurement applications, backlights create a black silhouette behind the part of product to provide ample contrast and clear edges.
Web inspection: In web inspection applications such as paper or textile inspection, a system must continuously inspect fast-moving materials. These applications typically use line scan cameras, which require specialized line scan lights that deliver a thin line of intensely bright light with high frequency to avoid flickering.
Scan tunnels: Scan tunnel systems deployed in logistics and warehousing facilities read barcodes at high speeds to deliver track and trace capabilities while increasing sorting and inbound/outbound operations throughput. While some tunnels may deploy barcode readers with built-in illumination, camera-based scan tunnels require high-powered bar/linear lights to illuminate boxes or parcels moving at high speeds.
More complex or specialized machine vision systems also require specific lighting implementations. For example, flexible cobot-based inspections can use a multi-functional light to avoid having to change over lights in between inspection types (Figure 2), while food inspection applications may require shortwave infrared, washdown lights, or both. No matter the application, all machine vision systems require proper lighting, including deep learning.
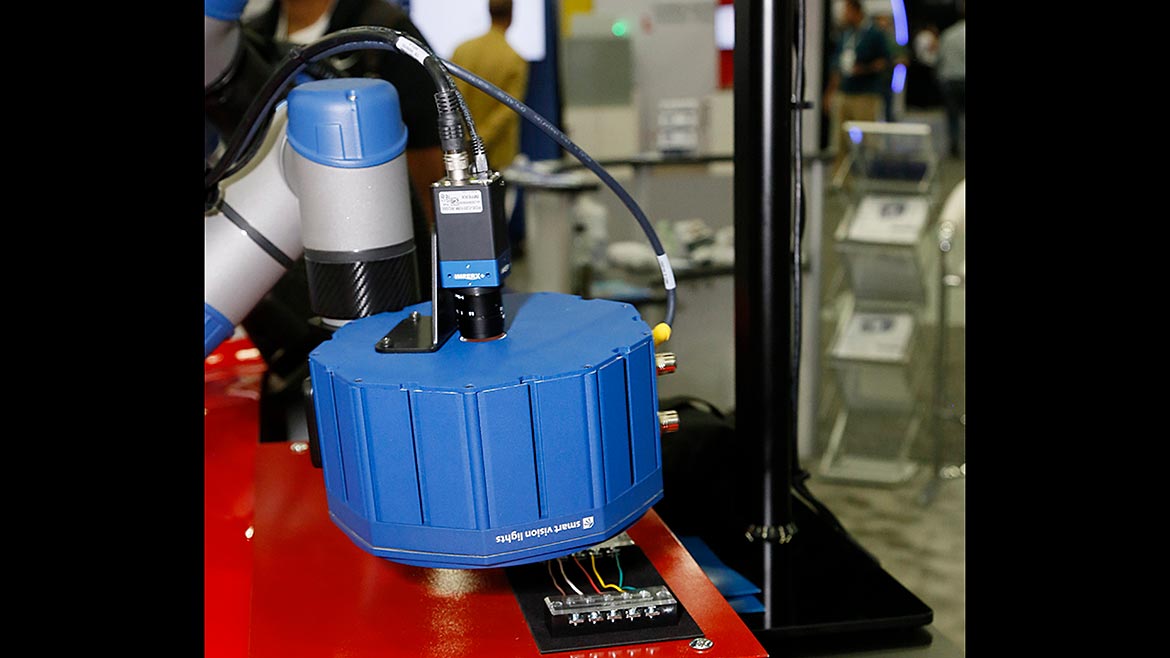
Bad Lighting Breeds Bad AI
Deep learning today is a powerful tool that has found success in many machine vision applications. The technology can help fill the gap where rules-based algorithms cannot suffice, in areas including optical character recognition, assembly verification, image classification, defect detection, and more. Finding success in a deep learning application involves a thorough application analysis and proper system setup. It is said that if just 10% of the data in a deep learning model is inaccurate, optimizing the model after the fact involves three times more data. It is critically important, as a result, to ensure that a data set comprised of high-quality images is used to program the model. As a result, once again, an optimal lighting setup is required.
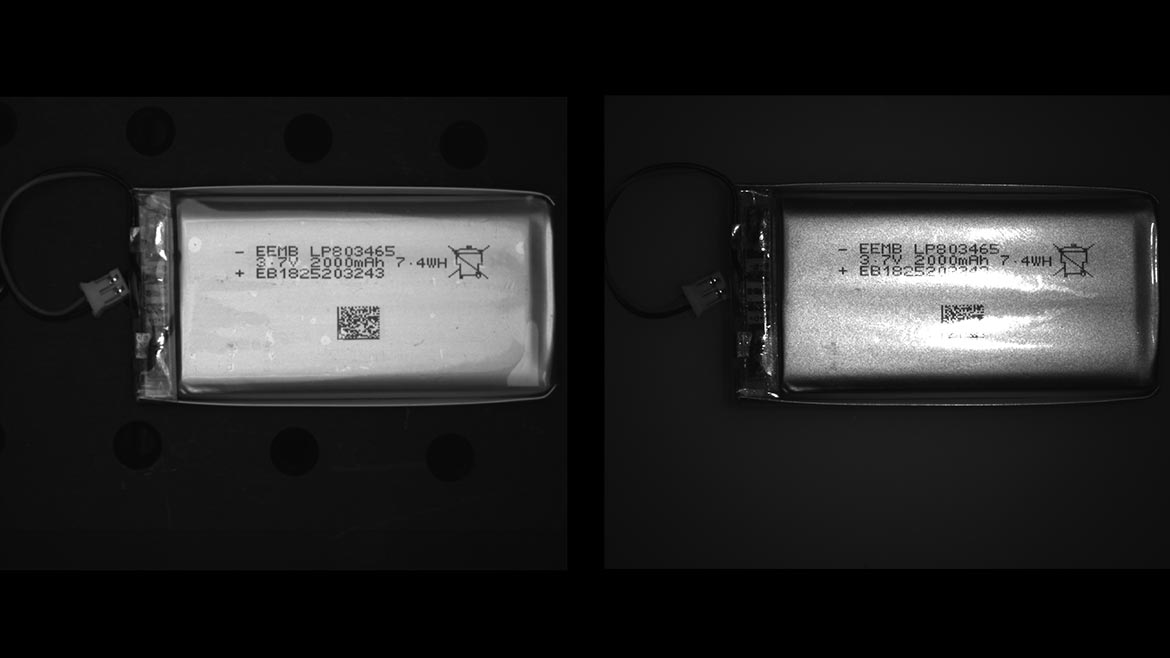
Incorrect lighting puts the reliability and success of a system at risk, and machine vision cameras and software algorithms cannot make up for inadequate illumination techniques in any type of application, even deep learning. Cliché as it may be by now, “garbage in, garbage out” still rings true. If you expect to train a deep learning model on bad images, the deep learning solution will not work. Simply relying on ambient illumination and not matching the correct lighting setup to the application can lead to light pollution, poor quality images, and the “garbage” referred to in the popular adage.
Selecting the right lighting for an application comes down to understanding what the system needs to capture, inspect, or detect. Different lighting setups will produce vastly different images, so the lighting and resulting effects must match the need of the application. Poor lighting configurations will produce poor feature extraction and poor deep learning models, which will lead to confusion on what is and isn’t a defect, or the identity of an object or feature.
Ultimately, different light means different images. Figure 4 shows the same spark plug gap tool, but with different lighting setups: front lighting, diffuse lighting, bright field lighting, dark field lighting, low angle dark field lighting, and backlighting. While the backlight image works great for measuring the diameter of the tool, for example, this would be virtually unusable for a surface defect detection application.
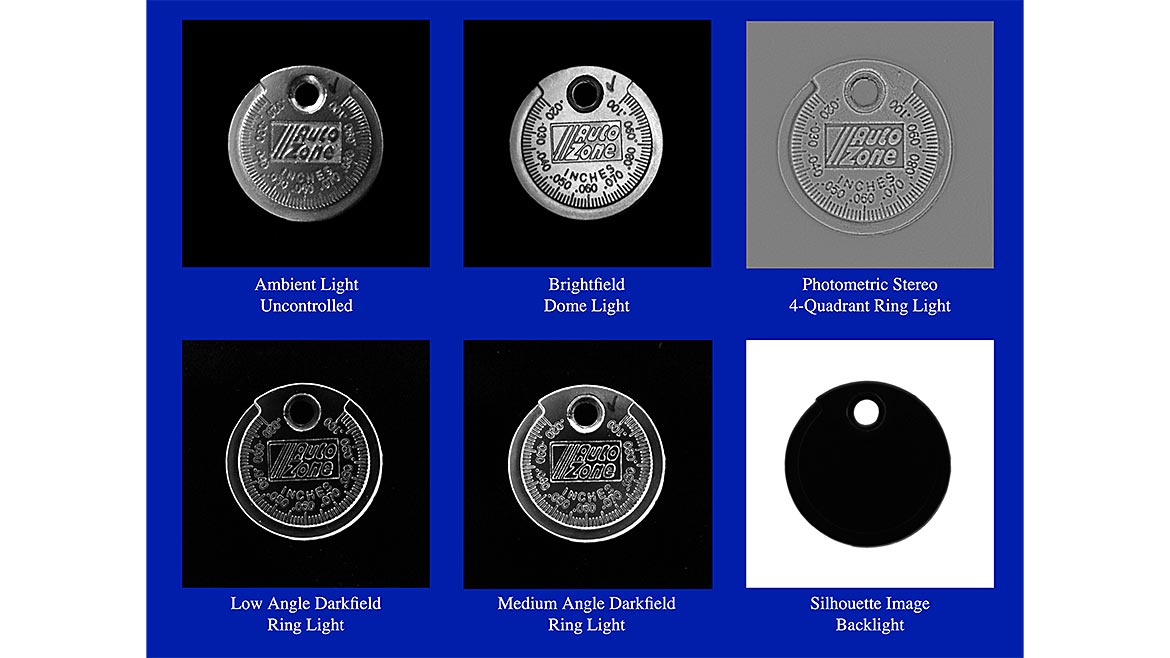
When it comes to developing a successful deep learning application, human expertise plays a significant role as well. There are ultimately no algorithms that can overcome bad lighting, so work smarter, not harder by focusing on the data. In this case, the data refers to high-quality images that have been labeled and categorized by human subject matter experts, because ultimately, deep learning software creates a mathematical model with data on extremely small image variations and other indicators that human experts use to determine if a part or product passes or fails. The model can then inspect new parts or products for quality, and learn over time like humans do, which is gaining new knowledge from experience and repeating successful operations. Essentially, one must ensure that the data clearly shows the AI what it needs to learn, and despite the rhetoric out there today, an optimal lighting setup is required for this.
Don’t Leave Lighting for Last
When it comes to developing a machine vision system with or without deep learning software, the requirements for creating high-quality images remain the same from a lighting perspective. Choosing the correct light involves a number of different considerations. Factors are dependent on individual applications, but typically include light structure, position, diffusion, and color. Most often, an application will require a lighting setup that maximizes contrast between features of interest and the background that is consistent, while other applications will require different lighting setups.
No matter the application, don’t wait until the end of the design process to consider lighting, which could lead to physical limitations in the system design. Instead, consider the desired vision system or deep learning outcomes from the start, and ensure that lighting is installed at the correct position relative to the camera. In all cases, remember that optimal lighting is irrefutably required for achieving success with machine vision and deep learning systems.